5.1 Effect Size
Figure 5.1: Sampling distribution of average candy weight under the null hypothesis that average candy weight is 2.8 grams in the population.
We have learned that larger samples have smaller standard errors (Section 3.3.1). Smaller standard errors yield larger test statistic values and larger test statistics have smaller p values. In other words, a test on a larger sample is more often statistically significant.
A larger sample offers more precision, so the difference between our sample outcome and the hypothesized value is more often sufficient to reject the null hypothesis. For example, we would reject the null hypothesis that average candy weight is 2.8 grams in the population if average weight in our sample bag is 2.70 grams and our sample is large. But we may not reject this null hypothesis if we have the same outcome in a small sample bag.
The larger our sample, the more sensitive our test will be, so we will get statistically significant results more often. If we think of our statistical test as a security metal detector, a more sensitive detector will go off more often.
Of course, the size of the difference between our sample outcome and the hypothesized population value matters as well. This difference is called observed effect size. If average candy weight in our sample bag deviates more from the average weight specified in the null hypothesis, we are more likely to reject the null hypothesis. In terms of a security metal detector: Our test will pick up large pieces of metal more easily than small pieces.
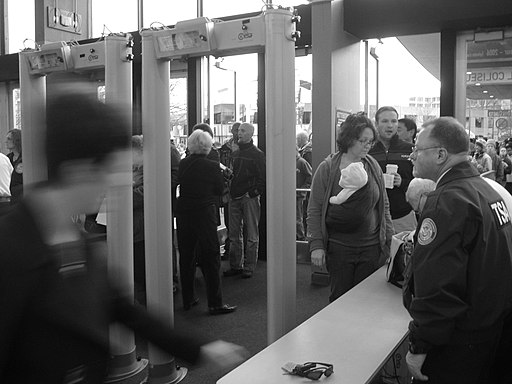
The p value and rejection of the null hypothesis based on the p value, then, depend both on sample size and observed effect size.
A larger sample size makes a statistical test more sensitive. The test will pick up (be statistically significant for) smaller effect sizes.
A larger effect size is more easily picked up by a statistical test. Larger effect sizes yield statistically significant results more easily, so they require smaller samples.
Deciding on our sample size, we should ask ourselves this question: What effect size should produce a significant test result? In the security metal detector example, at what minimum quantity of metal should the alert sound? To answer this question, we should consider the practical aims and context of our research.
5.1.1 Practical relevance
Investigating the effects of a new medicine on a person’s health, we may require some minimum level of health improvement to make the new medicine worthwhile medically or economically. If a particular level of improvement is clinically important, it is practically relevant (sometimes called practically significant).
If we have decided on a minimum level of improvement that is relevant to us, we want our test to be statistically significant if the average true health improvement in the population is at least of this size. We want to reject the null hypothesis of no improvement in this situation.
For media interventions such as health, political, or advertisement campaigns, one could think of a minimum change of attitude affected by the campaign in relation to campaign costs. A choice between different campaigns could be based on their efficiency in terms of attitudinal change per cost unit.
Note the important difference between practical relevance and statistical significance. Practical relevance is what we are interested in. If the new medicine is sufficiently effective, we want our statistical test to signal it. In the security metal detector example: If a person carries too much metal, we want the detector to pick it up.
Statistical significance is just a tool that we use to signal practically relevant effects. Statistical significance is not meaningful in itself. For example, we do not want to have a security detector responding to a minimal quantity of metal in a person’s dental filling. Statistical significance is important only if it signals practical relevance. We will return to this topic in Chapter 6.
5.1.3 Standardized effect size and sample size
We can use standardized effect size to express the effects that we are interested in. We choose whether small, moderate, or large effects are of practical interest to us. Preferably, we know from previous research whether small, moderate, or large effects are common in our type of research. If moderate or large effects are rare, we should use a sample size that allows detecting small effects. In contrast, when large effects occur frequently, we can do with a smaller sample that may overlook small effects.
If we know the effect size in the sample for which we want statistically significant results, we can figure out the minimum sample size for which the test statistic is statistically significant.
Figure 5.2: What is the minimum sample size required for a significant two-sided test result if the sample mean has a particular effect size? The p values belong to two-sided tests.
Observed effect size as well as test statistics reflect the difference between what we expect according to the null hypothesis and what we observe in our sample. As a consequence, effect size indicators and test statistics are related. In some cases, such as Cohen’s d, the relation between effect size and test statistic is very simple.
The test statistic t for a t test on one mean, for example, is equal to Cohen’s d times the square root of sample size. Here, the only difference between the two is sample size! Sample size influences the test statistic—the larger the sample, the larger the test statistic— but it does not influence effect size. This is an important difference.