Chapter 11 Mediation with Regression Analysis
Key concepts: causality, causal and time order, common cause, direct, indirect, and total effects, causal model, path diagram and path model, parallel and serial mediation, partial mediation, covariate, controlling mediator values.
Watch this micro lecture on mediation for an overview of the chapter.
Summary
If we add a causal order among the predictors of our regression model, we obtain a causal model or path model. The causal model includes an indirect effect: The first predictor affects the scores on the second predictor, which affects the scores on the dependent variable that we usually call the outcome variable. For example, age affects news site use, which affects newspaper reading time. In this path model, the second predictor (news site use) mediates the effect of the first predictor on the outcome variable. The second predictor is called a mediator.
We can estimate a path model as a series of regression models. The size of an indirect effect equals the product of the direct effects that constitute the indirect effect. With additional software, we can estimate the confidence interval of an indirect effect.
Mediation models help us to think about the different ways in which a variable may cause another variable. But we must realize that the causal order underlying the model is an assumption that we make. A regression model shows the predictive effects, which do not have to be causal. We cannot prove that the predictive effects are causal. We can only think of arguments that make a causal interpretation of a predictive effect plausible.
Essential Analytics
We can analyze a mediation model with a series of regression analyses in SPSS (the Linear option in the Regression submenu). We need one regression model for each variable that is predicted by one or more other variables in the model. The predicted variable is the dependent variable and as independent variables we use all variables that we think are causally prior to the dependent variable.
As an alternative, we may use the PROCESS macro for SPSS. The macro requires that we distinguish between parallel mediation (each indirect effect has just one mediator) and serial mediation (at least one indirect effect includes two or more mediators). PROCESS gives us the size and bootstrapped confidence intervals for all indirect effects of the selected predictor via mediators on the final outcome variable.
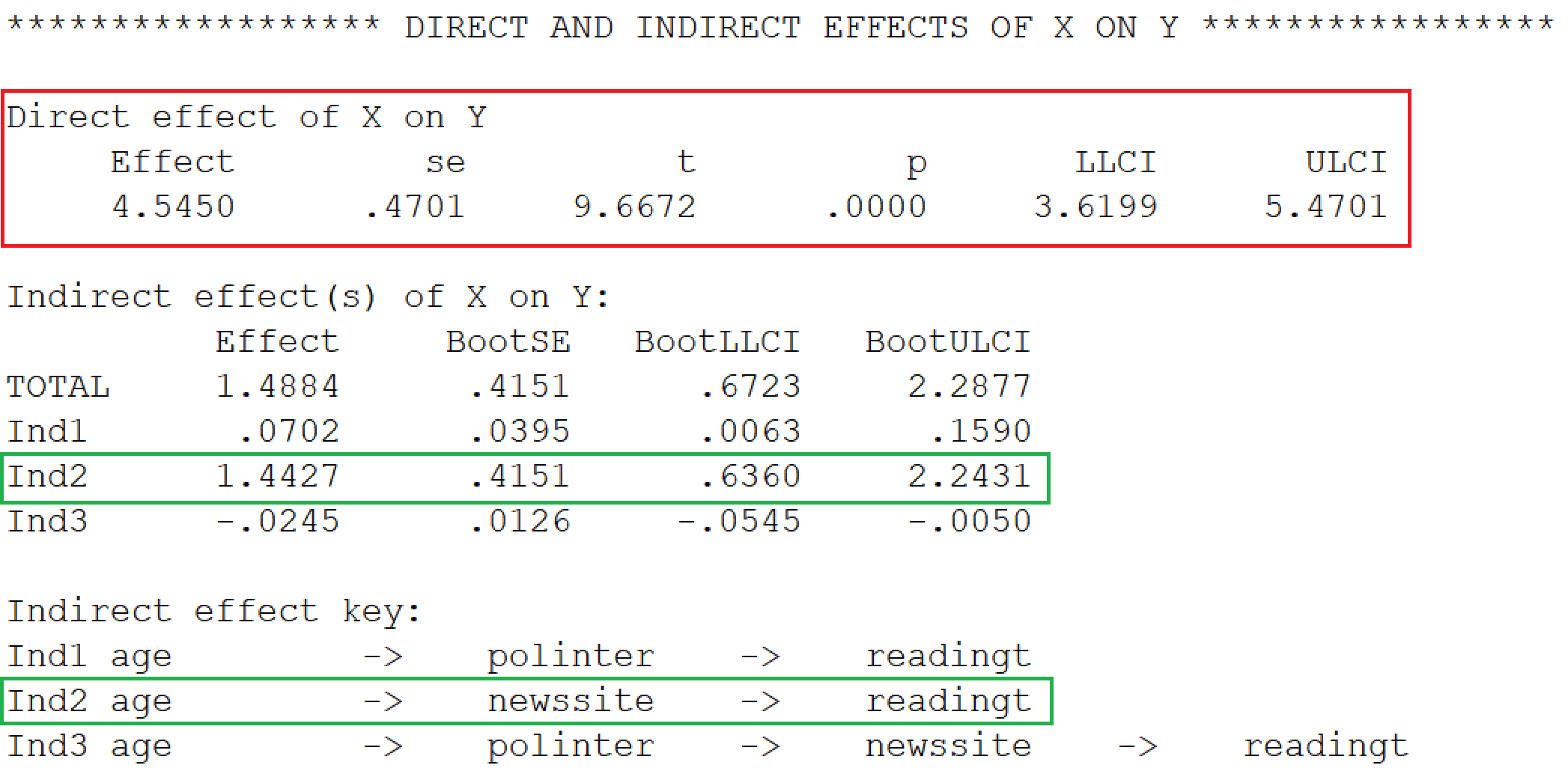
Figure 11.1: PROCESS output with estimated indirect and total effects of age on average newspaper reading time.
Figure 11.1 shows the results of a model in which political interest and news site use mediate the effect of age on newspaper reading time. Apart from a direct effect (b = 4.55, 95%CI[3.62, 5.47], red box in Figure 11.1), three indirect effects have been estimated. Clearly the strongest indirect effect of age is the effect mediated by news site use (Figure 11.1, green boxes). An additional unit of age increases predicted newspaper reading time by 1.44. The 95% confidence interval (95%CI[0.64, 2.24], bootstrapped) does not include zero, so this indirect effect is statistically significant at the .05 significance level.