10.3 Controlling for Covariates
We usually have theoretical reasons to expect that a variable mediates an effect, for example, that political interest mediates the effect of age on newspaper reading time. At the same time, we know that our dependent variable and perhaps our mediator may depend on other variables. Newspaper reading time, for instance, may also depend on education. In this situation, we would use the other variables as covariates (or control variables) for which we want to control statistically.
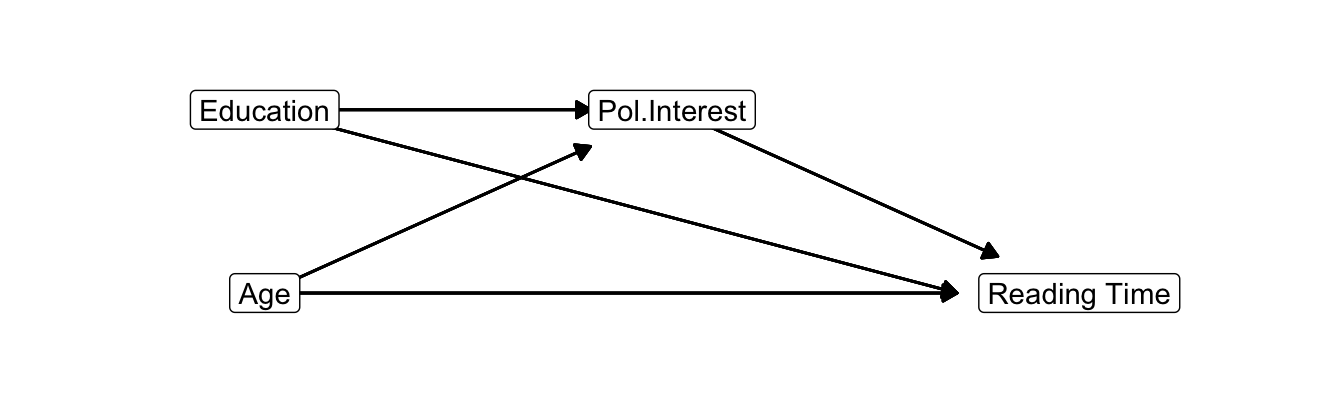
Figure 10.10: Causal diagram for interest in politics as mediator between age and newspaper reading time with education as covariate.
Figure 10.10 presents a model in which education is used as a covariate in a model with political interest mediating the effect of age on newspaper reading time. Education is probably causally prior to both political interest and newspaper reading time, so it is allowed to have an effect on both variables. In this way, we control for education and remove spurious correlation between political interest and newspaper reading time due to education as a common cause.
If education predicts political interest and political interest predicts newspaper reading time as in Figure 10.10, political interest mediates the effect of education on newspaper reading time. We are, however, not interested in mediation in the case of a covariate, so we do not estimate or report the indirect effects of education on newspaper reading time. In the context of a mediation model, a covariate is a predictor for which we do not investigate if its effect is mediated.
Note that covariates should only be allowed to have an effect on variables that can be caused by the covariate. We should not include effects of a covariate on a variable that is causally prior to it. If a covariate is a consequence rather than a cause of a mediator, it had better be used as another mediator in the model. If, for instance, political cynicism may affect newspaper reading time but it is a consequence of political interest, it should be included as a second (serial) mediator instead of as a covariate.